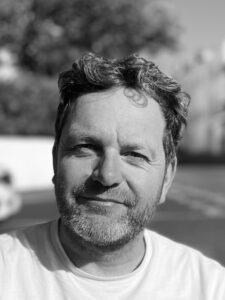
Artificial intelligence (AI) and machine learning (ML) are being applied to achieve significant efficiency gains across capital markets. One of the people who is deeply involved in the advancements of these technologies in our industry is Andy Simpson, Founder and CEO of financial markets analytics firm Sigma Financial AI. In the lead-up to our recent TT Connect: Unlocking Profit with Data and Analytics event, where he moderated the panel “AI – evolution or revolution?”, we caught up with Andy about how the growth of AI and ML is changing research and analytics.
What is Sigma Financial AI doing in data analytics?
We are a real time financial market analytics firm. Our view is that the world is changing from one in which data is analyzed using batch data at the end of the day to one which enables immediacy and personalization.
Today, market analytics lag what has happened by some distance. A trader can get research that analyzes what happened yesterday in Microsoft or Apple stocks or short-term interest rates futures. What that research can’t tell you is what is happening right now and why. Data analytics should be more immediate than the day after the event.
The advances enabled by AI mean that data analytics can deliver information about what’s happening in the market right now. It means that analytics can be responsive to market events in real time.
Our aim is to offer the level of responsiveness and personalization in which a trader can get the analysis and the research that’s of interest to them. These are the two themes that we are focused on as a business: personalization and immediacy of analytics, so that traders are not consuming stale analytics that don’t reflect their interests.
What have been the main challenges in building the platform?
The biggest challenge with any AI build is good quality data. But, in addition, for large language models to be effective, they need a golden source of good-quality data. We are instrument agnostic and in fact data agnostic. Add to that, we want to ensure our users have the responsiveness of a streaming data platform. This all requires significant engineering to process and surface that data in almost real time.
The recent evolution of AI has been driven by new technologies and an increasing abundance of processing power offered by cloud computing providers over the last decade. This paired with breakthrough techniques in the field has heralded a new wave of applications that would have been unattainable previously due to computing cost. The advent of cloud technology has been a real kicker and enabled new business models such as SaaS, and this in turn has driven new practices, which has helped increase innovation and decrease cost.
Because of the changes in technology and the increases in processing power that are available, the time to market is much shorter and the cost of delivery far, far smaller — possibly a 10th of the cost of what it would have been had we built this a decade ago.
How has the regulatory landscape for developing AI changed, and has that been a barrier or an enabler?
The regulatory landscape has been slow to develop around AI. You could consider that slow development an enabler for firms like ours though. It means that companies across the market are cautious when embarking upon large-scale AI projects. They’re nervous because they know they’ve got to skill up their team and spend time and money developing a project with the risk that regulatory oversight prevents them from launching.
If that was the case – they have spent all this money only to find that the sandbox doesn’t support them, or the regulatory architecture doesn’t support what they’ve built. The whole R&D cycle is very difficult in this environment. However, firms like ours can offer a low-risk means of starting an AI journey.
Regulation is now coming to govern AI, but regulators need to take a pragmatic approach. In the EU, the Digital Operational Resilience Act (DORA) regulation will support technology implementation and outsourcing in a comprehensive and well-understood way, creating a clear regulatory architecture. I don’t think regulators need to re-engineer all of that just for AI.
AI is not new. We have been using artificial intelligence for 30 or 40 years in various forms of computing. It’s only since large language models have come about that people have started to raise concerns. My view of AI is that it has been in existence for a long time, longer than people realize, and therefore we do have the skills and capabilities to put in place frameworks to make sure we can support the ongoing innovation while maintaining a safe environment for development.
How have you found the availability of funding for AI projects in Europe compared with the U.S.?
I think that the economic situation here is improving now and we are seeing a lot more appetite for seed funding for AI-based projects, but we are still far away from the U.S. There is less risk-taking in the European and UK mindset, and that has been dampened further because of recent economic cycles.
It’s been quite a challenging environment for fundraising in Europe for AI startups. The U.S. is far more interested in developing R&D ideas, which is reflected in the fact that our first customers have been in the U.S., but now we are starting to see traction in the UK.
What is the future of AI in analytics?
I think that over the next two or three years, AI will become embedded to the extent that it is a fundamental part of how firms do business. At the same time, there is the ongoing increase in the compute power enabling large language models, machine learning and new themes such as continuous learning.
That additional capacity has enabled us to do far greater data analysis than we ever were able to do previously. So, if you fast forward two or three years, there’s every reason to think that compute power will continue to increase and the costs will continue to come down.
The other angle is, of course, that we get data squared. The more data we have and the more data we analyze, the more data we create. And so storage capacity and cost will become increasingly important.
But I genuinely believe that personalization and immediacy will be the real trends that drive analytics over the next decade. I think we will continue to see this trajectory: the ability to offer personalization and immediacy at a scale we’ve never been able to achieve before.